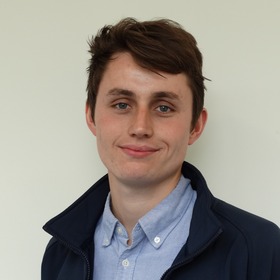
Dr Tally Wright, NIAB, UK
Tally is leading a group in quantitative genetics within the pre-breeding department at NIAB. The group explores statistical genetics, experimental design, and diverse methods of data analysis. NIAB is the UK’s fastest growing crop science organisation, with rapidly expanding research capabilities in plant genetics, agronomy, farming systems and data science, the largest national field trials capability and strong research links with industry, government and academia. At NIAB, Tally works across a range of ongoing research projects in Cambridge Crop Research division and is involved in the analysis and design of a number of diverse and important NIAB wheat collections.
Before his current position, Tally worked as a postdoctoral researcher exploring genetic data analysis in the NIAB Genetics and Breeding group. In this role he was responsible for contributing to ongoing projects that generated large genetic data sets for gene discovery (e.g. QTL mapping, especially in multi-founder populations, GWAS), screening of genetic resources, crop genomics, and genomic selection. Tally also has a background in crop physiology, which was the focus of his PhD obtained from the University of Cambridge in 2018. He studied under a funded BBSRC PhD studentship built around the theme of ‘Agriculture and Food Security’. Tally’s studentship was based at NIAB, where he examined photosynthetic diversity in wild wheat species and how this diversity can be harnessed to improve modern wheat.
Introduction to Field Trials Workshop – 11th October 2022
Title: Utilising relevant experimental design and statistics to further your research in the field, glasshouse or growth chamber.
Abstract: Whether your experiments are in the growth chamber, glasshouse or field, elements outside of our control can confound the data collected, leading to misinterpretation of results. These confounding and nuisance factors can easily be controlled through appropriate experimental design. While these skills are an essential tool for every scientist, it is something often overlooked. By taking advantage of homogeneity within experimental locations, block designs are frequently used to reduce field fertility effects. When designing these experiments, it is important to consider the downstream statistical techniques that will used to interpret the data. Linear mixed models are powerful tools that, in addition to residual error, include fixed factors that we are interested in (e.g. genotype or treatment) and random factors that we may not be interested in (e.g. blocking or location). With these methods, we can improve predictions or estimates of variety means for more accurate genetic mapping, genomic prediction, comparisons between genotypes and exploring genotype x environment interactions. Thanks to available statistical resources, understanding the underlying mathematical theory behind these techniques is not required. However, scientists still need a good grasp of the concepts to avoid pitfalls and to aid correct interpretation of their results. From a biologist’s point of view, this talk will summarise the basics of trial design and analysis, while detailing resources available for further exploration of these techniques in the user’s own research.